Join our daily and weekly newsletters for the latest updates and exclusive content on industry-leading AI coverage. Learn More
Another day, another announcement about AI agents.
Hailed by various market research reports as the big tech trend in 2025 — especially in the enterprise — it seems we can’t go more than 12 hours or so without the debut of another way to make, orchestrate (link together), or otherwise optimize purpose-built AI tools and workflows designed to handle routine white collar work.
Yet Emergence AI, a startup founded by former IBM Research veterans and which late last year debuted its own, cross-platform AI agent orchestration framework, is out with something novel from all the rest: an AI agent creation platform that lets the human user specify what work they are trying to accomplish via text prompts, and then turns it over to AI models to create the agents they believe are necessary to accomplish said work.
This new system is literally a no code, natural language, AI-powered multi-agent builder, and it works in real time. Emergence AI describes it as a milestone in recursive intelligence, aims to simplify and accelerate complex data workflows for enterprise users.
“Recursive intelligence paves the path for agents to create agents,” said Satya Nitta, co-founder and CEO of Emergence AI. “Our systems allow creativity and intelligence to scale fluidly, without human bottlenecks, but always within human-defined boundaries.”
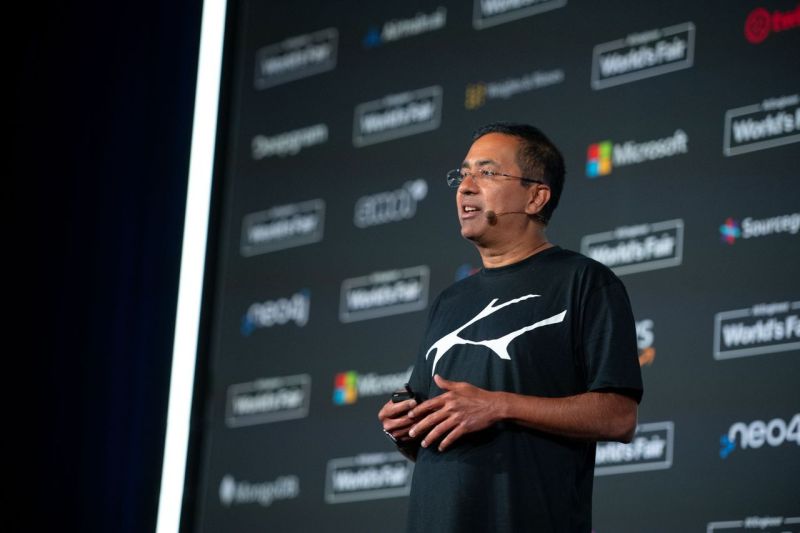
The platform is designed to evaluate incoming tasks, check its existing agent registry, and, if necessary, autonomously generate new agents tailored to fulfill specific enterprise needs. It can also proactively create agent variants to anticipate related tasks, broadening its problem-solving capabilities over time.
According to Nitta, the orchestrator’s architecture enables entirely new levels of autonomy in enterprise automation. “Our orchestrator stitches multiple agents together autonomously to create multi-agent systems without human coding. If it doesn’t have an agent for a task, it will auto-generate one and even self-play to learn related tasks by creating new agents itself,” he explained.
A brief demo shown to VentureBeat over a video call last week appeared duly impressive, with Nitta showing how a simple text instruction to have the AI categorize email sparked a wave of new agents being created, displayed on a visual timeline showing each agent represented as a colored dot in a column designating the category of work it was designed to help carry out.

Nitta also said the user could stop and intervene in this process, conveying additional text instructions, at any time.
Bringing agentic coding to enterprise workflows
Emergence AI’s technology focuses on automating data-centric enterprise workflows such as ETL pipeline creation, data migration, transformation, and analysis. The platform’s agents are equipped with agentic loops, long-term memory, and self-improvement abilities through planning, verification, and self-play. This enables the system to not only complete individual tasks but also understand and navigate surrounding task spaces for adjacent use cases.
“We’re in a weird time in the development of technology and our society. We now have AI joining meetings,” Nitta said. “But beyond that, one of the most exciting things that’s happened in AI over the last two, three years is that large language models are producing code. They’re getting better, but they’re probabilistic systems. The code might not always be perfect, and they don’t execute, verify, or correct it.”
Emergence AI’s platform seeks to fill that gap by integrating large language models’ code-generation abilities with autonomous agent technology. “We’re marrying LLMs’ code generation capabilities with autonomous agent technology,” Nitta added. “Agentic coding has enormous implications and will be the story of the next year and the next several years. The disruption is profound.”
Emergence AI highlights the platform’s ability to integrate with leading AI models such as OpenAI’s GPT-4o and GPT-4.5, Anthropic’s Claude 3.7 Sonnet, and Meta’s Llama 3.3, as well as frameworks like LangChain, Crew AI, and Microsoft Autogen.
The emphasis is on interoperability—allowing enterprises to bring their own models and third-party agents into the platform.
Expanding multi-agent capabilities
With the current release, the platform expands to include connector agents and data and text intelligence agents, allowing enterprises to build more complex systems without writing manual code.
The orchestrator’s ability to evaluate its own limitations and take action is central to Emergence’s approach.
“A very non-trivial thing that’s happening is when a new task comes in, the orchestrator figures out if it can solve the task by checking the registry of existing agents,” Nitta said. “If it can’t, it creates a new agent and registers it.”
He added that this process is not simply reactive, but generative. “The orchestrator is not just creating agents; it’s creating goals for itself. It says, ‘I can’t solve this task, so I will create a goal to make a new agent.’ That’s what’s truly exciting.”
Bet lest you worry the orchestrator will spiral out of control and create too many needless custom agents for each new task, Emergence’s research on its platform shows that it has been designed to — and successfully carries out — the additional requirement of winnowing down the number of agents created as it comes closer and closer to completing a task, adding agents with more general applicability to its internal registry for your enterprise, and checking back with that before creating any new ones.

Prioritizing safety, verification, and human oversight
To maintain oversight and ensure responsible use, Emergence AI incorporates several safety and compliance features. These include guardrails and access controls, verification rubrics to evaluate agent performance, and human-in-the-loop oversight to validate key decisions.
Nitta emphasized that human oversight remains a key component of the platform. “A human in the loop is still important,” he said. “You need to verify that the multi-agent system or the new agents spawned are doing the task you want and went in the right direction.” The company has structured the platform with clear checkpoints and verification layers to ensure that enterprises retain control and visibility over automated processes.
While pricing information has not been disclosed, Emergence AI invites enterprises to contact them directly for access and pricing details. Additionally, the company plans a further update in May 2025, which will extend the platform’s capabilities to support containerized deployment in any cloud environment and allow expanded agent creation through self-play.
Looking ahead: scaling enterprise automation
Emergence AI is headquartered in New York, with offices in California, Spain, and India. The company’s leadership and engineering team include alumni from AI research labs and technology teams at IBM Research, Google Brain, The Allen Institute for AI, Amazon, and Meta.
Emergence AI describes its work as still in the early stages but believes its recursive intelligence approach could unlock new possibilities for enterprise automation and, eventually, broader AI-driven systems.
“We think agentic layers will always be necessary,” Nitta said. “Even as models get more powerful, generalization in the action space is incredibly hard. There’s plenty of room for people like us to advance this over the next decade.”
READ SOURCE